Early identification of dropout risks, follow-up study (FragSte 2)
Project outline
In the BMBF-funded project FragSte, an early warning system for predicting impending dropouts was developed in 2017 until 2020. With FragSte, potential university dropouts are detected with the help of process data and machine learning methods. Students can be reached with automated feedback and the impact of feedback on dropouts can be tested. Accurate predictions are already possible and students at risk of dropping out can be offered help - but not before the middle of the second semester. Thus, interventions are only possible within the second exam period. Intervention successes will thus only be possible in the exam phase at the end of the third semester. This is too late. On the one hand, many students at risk of dropping out will have already left the university by then, and those who remain will have lost a lot of time. Another finding of the first project phase is that low-threshold information interventions for students at risk of dropping out are not effective. In the second funding period, FragSte will be further developed by integrating the approach and support services into teaching as well. In FragSte 2, three subprojects are planned:
- How can an early warning system at universities predict impending dropouts even during the first semester?
- Can dropouts be classified according to study motivation in a more differentiated way?
- How can students at risk of dropping out be better reached?
To answer these questions, in addition to the administrative data used so far at the universities, the registration behavior for exams and the learning behavior on learning platforms will be evaluated. Furthermore, a randomized experiment will test the effect of self-assessments with and without feedback as well as offers by teachers. The results will also be used to further sharpen dropout predictions and to classify dropouts more precisely. So far, dropouts have been considered as a binary variable. This means that mock students who do not pursue a degree are counted as dropouts in the same way as students with subject-specific deficits. However, as long as the proportion of mock students is not known, neither the need for action nor the effectiveness of existing measures can be adequately assessed.
Additional information
ongoing
2021 until 2024
FragSte, FragSte 2, drop-outs, course of studies, first semester, drop-out, monitoring, intervention
Kerstin Schneider (project lead)
Simon Görtz (project lead)
Johannes Berens (contact person)
J. Leandro Henao
Axel Schomaker
Franz Westermaier
- Schneider, K., Berens, J., Görtz, S. (2021): Automatisierte Früherkennung abbruchgefährdeter Studierender: Was können die Systeme leisten und was sind die Herausforderungen? Handbuch Qualität in Studium, Lehre und Forschung, Berlin, DUZ Verlags- und Medienhaus.
- Schneider, K., Berens, J., Görtz, S. (2021): Maschinelle Früherkennung abbruchgefärdeter Studierender und Wirksamkeit niedrigschwelliger Intervention. In Neugebauer, M., Daniel, H.D., Wolter, A. (Hrsg.): Studienerfolg und Studienabbruch. Wiesbaden, Springer VS.
- Berens, J., Schneider, K., Görtz, S., Oster, S., Burghoff, J. (2019): Early Detection of Students at Risk - Predicting Student Dropouts Using Administrative Student Data from German Universities and Machine Learning Methods. In: Journal of Educational Data Mining, 11(3), 1-41. https://doi.org/10.5281/zenodo.3594771
- Schneider, K., Berens, J., Burghoff, J. (2019): Drohende Studienabbrüche durch Frühwarnsysteme erkennen: Welche Informationen sind relevant?. In: Zeitschrift für Erziehungswissenschaften, 22, 1121-1146. DOI: 10.1007/s11618-019-00912-1
- Berens, J., Schneider, K. (2019): Drohender Studienabbruch: Wie gut sind Frühwarnsysteme? In: Qualität in der Wissenschaft (QiW), 3+4/19, 102-108. ISSN 1860-3041
- Berens, J., Schneider, K., Görtz, S., Oster, S., Burghoff, J. (2018): Early Detection of Students at Risk - Predicting Student Dropouts Using Administrative Student Data and Machine Learning Methods. CESifo Working Papers, 7259. [download from RePEc]
About FragSte2 (Video, German)
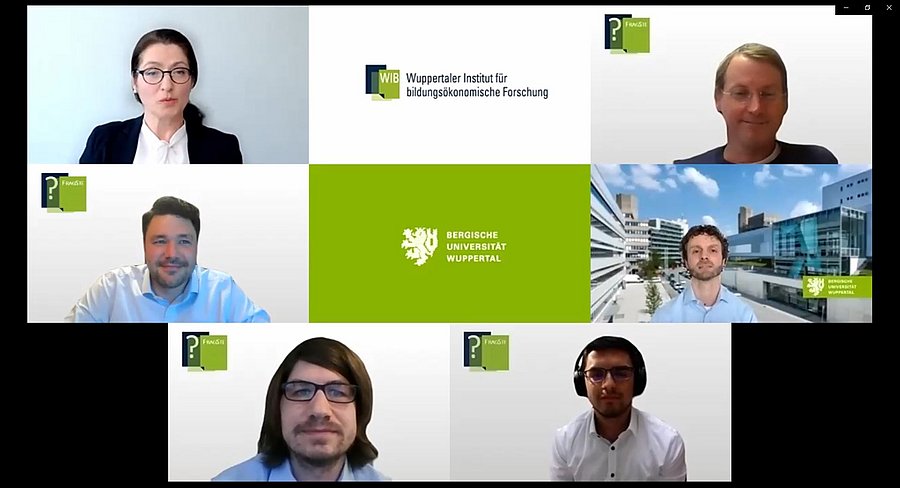
Start video
Read more about
Funded by:
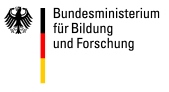